The Future of Data Dashboards
You are probably thinking: What does a car dashboard have to do with the future? And that's precisely the point of this article.
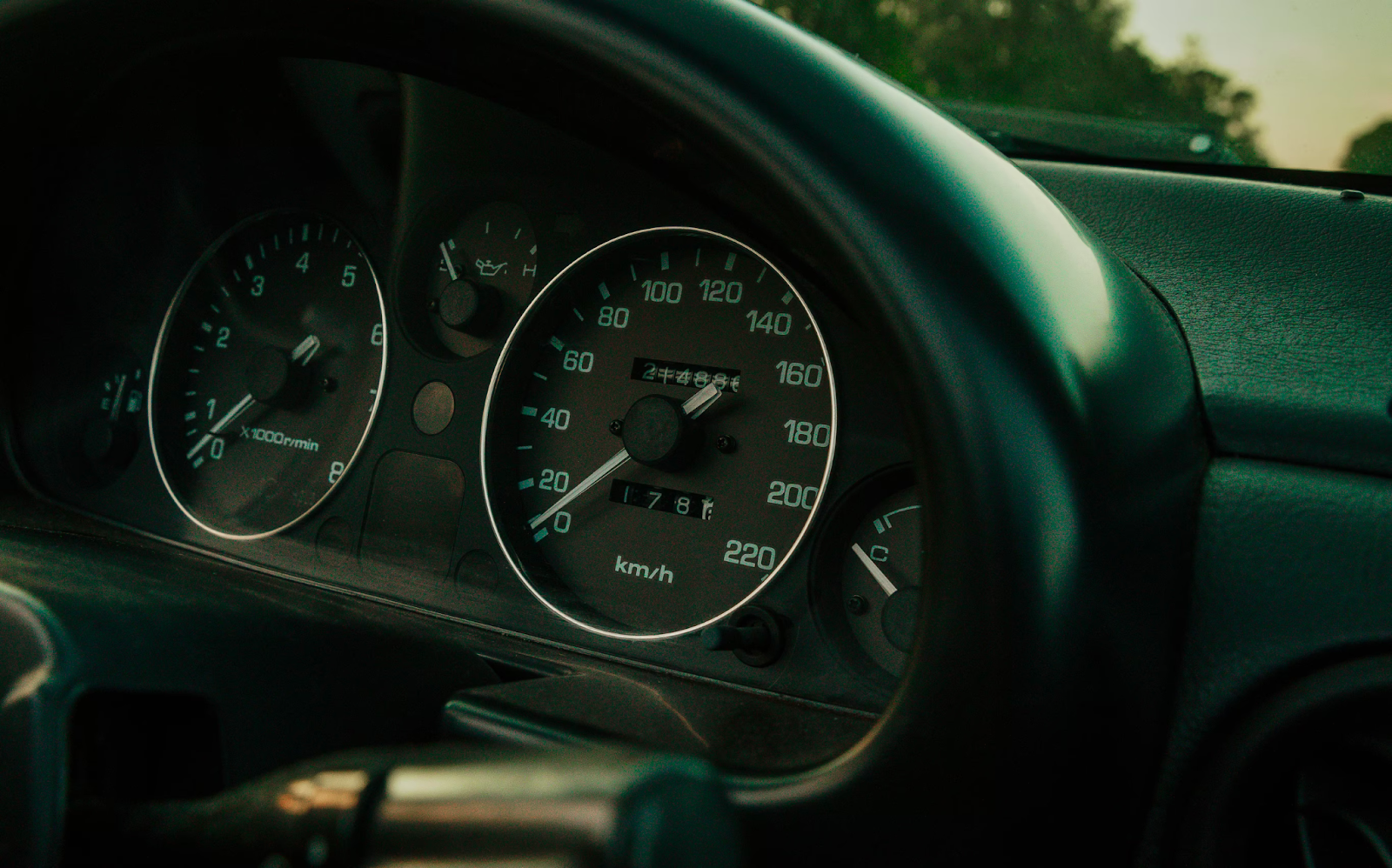
Data dashboards today have much in common with how car dashboards are designed. Everything critical to the driver is presented visually in a single place – How fast are we going? How much gas? What warnings are on?
While this design has served us well, I think the future will be nothing like it.
Let me take you on a ride.
Dead Dashboards are Everywhere
In the early days of conducting customer research for Toric, we met around 100 companies. We had to understand how data was communicated and how users felt about it. We would ask: "What do you consider a good dashboard?" to find leaders with a blank stare. We would then get shown dashboards with outdated data over 3+ months old.
So, why do dashboards die off?
Originally, the word dashboard applied to a barrier of wood or leather fixed at the front of a horse-drawn carriage or sleigh to protect the driver from mud or other debris "dashed up" (thrown up) by the horses' hooves.
Data Context and Trust
It's easy to imagine a future with a "single pane of glass" at which you can wave your hands, and data is available at your fingertips. There are countless movies to reference here.
There is just one problem: it lacks context — and data without context is hard to trust.
The reality is that interpreting vast amounts of data requires deep context. To have context about a piece of information is to know:
- Its meaning.
- The driving forces behind it.
- Its source.
- How it has changed over time.
Once you have context, it is possible to understand how it relates to other metrics and the impact on the overall system you are observing, which creates confidence and trust.
Car dashboards have context and are easy to trust. A fuel gauge is a direct measure of fuel – it is physically connected to the device providing the information, and it does so in real-time – but the real insight is derived. The amount of fuel doesn't tell you how far the car will go; it tells you how much fuel is left; the driver makes an approximation (based on the knowledge they have of their car fuel consumption per mile) to interpret that data point.
The impact of running out of gas is beyond obvious, and the decisions are simple. Because you understand the context of the fuel gauge, you trust the insights on mileage.
So does having access to more "gauges" increase context and, therefore, trust? Clearly, that's not the case for Tesla owners – they trust their cars, despite having an empty dashboard.
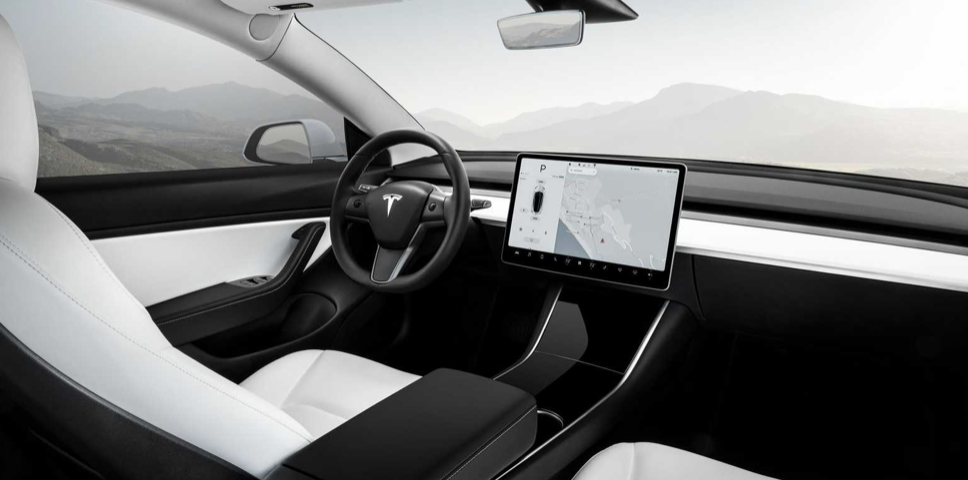
Having more information thrown at you does not equate to more trust – it's the belief that the data is accurate and that you understand its meaning that creates trust.
Why Do Data Dashboards Die ?
The moment of death for a dashboard is when it's brought up on the big screen, and someone points out information they don't trust. At that moment, the dashboard graveyard gods start fabricating a new coffin as trust dissipates and people pull up a spreadsheet to continue the meeting. Dashboards — being designed as one-way interaction models — in the end, kill collaboration around data.
In fact, most dashboards are glorified power points.
The problem is compounded as data complexity increases. At the beginning of a project, it is easy to update a dashboard. But then a few minutes quickly become hours or even days for someone to wrangle data into a presentation layer. And the less time you have to put invest, the sooner the dashboard is retired.
The other reason why it's so hard to maintain dashboards is because you need specialized domain knowledge to retrieve most of the data – imagine if you had to call the Toyota technician every time you wanted to know your car’s fuel level. This is what it looks like to build a dashboard. The people who need the information cannot easily retrieve it, and they need to call a specialist to read, transform and feed data into the dashboards.
Not All Data Is Born Equal
Dashboards that contain a lot of computed/derived data and many contexts become an engineering challenge. Now, instead of spending time using the information, the time is spent just making the dashboard work.
As an example, if we are building a dashboard for a sales team with goals/KPIs per quarter and previous quarter benchmarks. This is what we have:
- Obvious context: the data in a sales dashboard is directly derived from records in a database – just like the fuel gauge on a car dashboard.
- No indirections or computation is necessary – you are simply observing measurements.
- The decisions are straightforward – e.g., which customers to focus on for the quarter.
In fact, this data is so easy to leverage that most CRMs will have a dashboard built in!
Now, let's say you are observing an engineering team's performance. Or an architecture and construction project. You might want to know:
- An overall estimate of the cost to complete the work, based on project scope.
- The amount of work already completed, compared to project specification.
- Visibility to quantities of things such as mechanical parts, volumes, and areas in a building design.
This scenario is nothing like the sales team scenario. Here, there is way too much data to cope with at once, and many contexts are needed. Autodesk has estimated that decisions made with bad data cost $88.69 billion dollars to construction companies in 2020.
The hard truth is that most BI and data visualization solutions were built around sales, marketing, and financial reporting needs. We are living in a far more complex world now. So, where do we go from here?
The Future: Data Visualzation
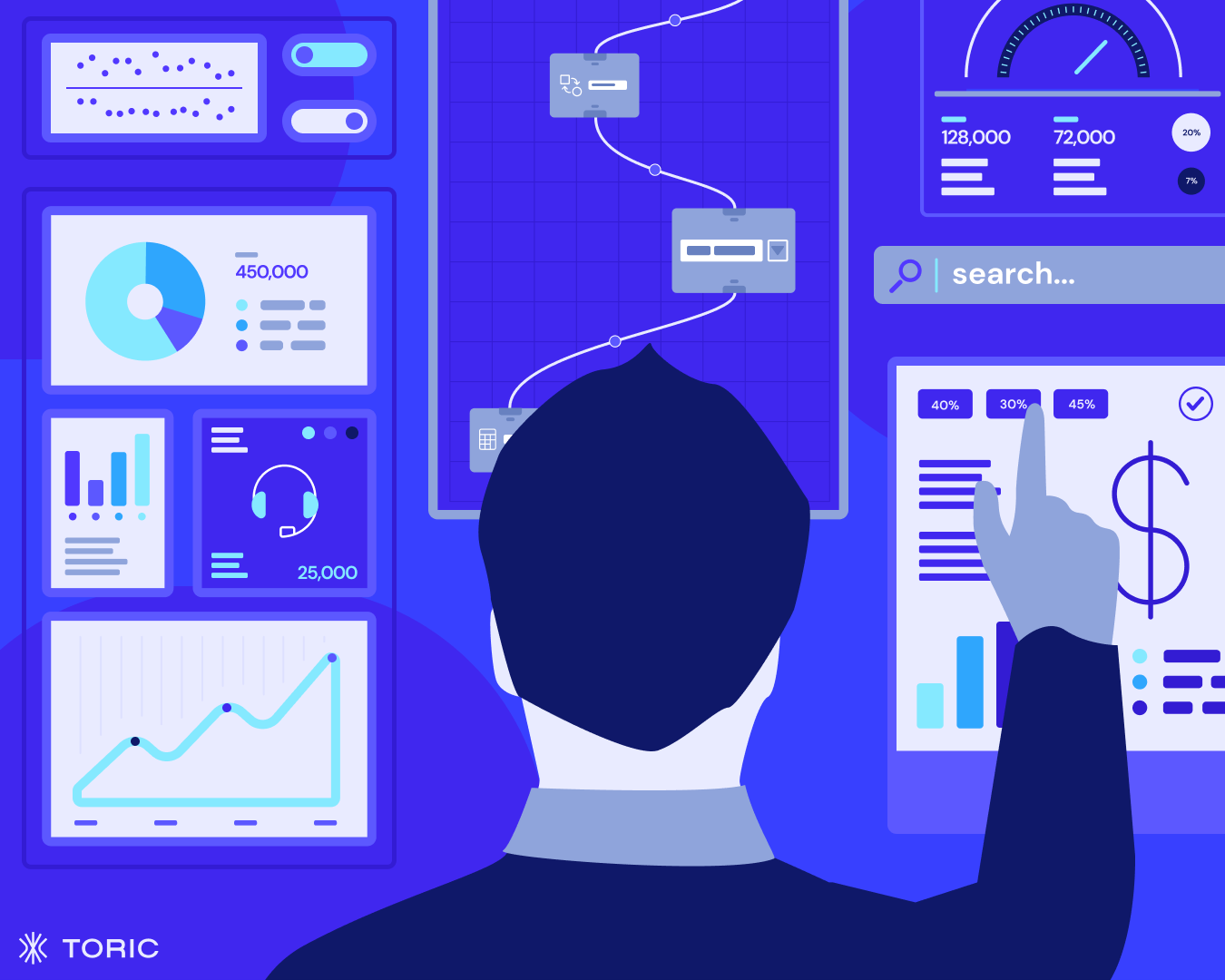
The very idea that you need to fit all your context, data, and insights into one fixed report page size is preposterous. And I think a lot of people are starting to see this as well.
Dashboards are morphing into different types of data consumption experiences:
- Data apps / data documents.
- Data notebooks.
- Embed-analytics.
- Mobile data-apps.
- Search analytics.
- Inbox analytics.
To power these new experiences, no code Data pipelines are becoming easier to build with no code — It's not feasible to develop and deploy software each time you must answer a new data question, so data transformation is gaining momentum as a first class problem.
The future I think we will see is one where more people in the organization can leverage data to make trustworthy decisions. And the repetitive work data people are doing will reduce as people will gain more time to focus on actual insights.